22. May 2025
How BLU DELTA Combines Modern AI Technology with OCR and Internal Data Sources
In the rapidly evolving world of artificial intelligence (AI), new approaches are emerging almost daily that offer real added value to businesses. One of the most promising technologies currently is Retrieval-Augmented Generation (RAG). At BLU DELTA, we are deeply engaged with RAG — and here we explain why this method is particularly relevant when combined with internal document processing and OCR.
What is Retrieval-Augmented Generation (RAG)?
RAG combines a Large Language Model (LLM) — such as GPT — with an external knowledge source. Unlike traditional language models that rely solely on their training data, RAG can dynamically incorporate additional context — for example, from internal documents or up-to-date information.
At the heart of RAG is semantic search within a vector-based database: the appropriate answer is generated based on both the user query and the most relevant content from an organization’s own knowledge base.
Why Aren’t Classic LLMs Enough?
LLMs are impressive in their ability to understand and generate natural language — but in everyday use, they often fall short:
-
-
No access to current or internal data
-
Limited context window — longer or complex content may be lost
-
No source citations, making trust and traceability more difficult
-
Hallucinations — the model may “invent” facts when lacking relevant information
-
This becomes a real issue for companies wanting automated access to invoices, contracts, or policies. This is where RAG comes in — ideally in combination with OCR technologies, which make documents like PDFs or scanned receipts searchable.
How Does RAG Work in Practice?
RAG consists of three core steps:
- Indexing: Internal documents — whether digital or extracted via OCR — are split into small, structured units ("chunking") and stored in a vector database.
- Retrieval: When a question is asked, a semantic algorithm searches the database and identifies relevant text passages.
- Generation: The LLM receives both the question and the retrieved snippets as context, and generates a precise answer — complete with a source reference.
This enables questions about internal data, policies, or contract details to be answered directly and transparently.
Advantages and Potential of RAG
RAG offers a wide range of benefits:
- Up-to-date information: New data can be added without retraining the model
- Transparency: Every answer includes a source reference
- Data security: Company knowledge remains internal; the LLM only accesses prepared contexts
- Scalability: Quick integration into existing workflows — e.g., via the MCP Server
- OCR integration: Even physical or scanned documents become part of the AI evaluation through text recognition
RAG combines the strengths of LLMs with your organization’s knowledge.
Challenges? Of Course — But Solvable
RAG isn’t a plug-and-play solution. Quality depends on a well-thought-out chunking strategy and a precise retriever. Evaluating the generated answers also requires care — especially in sensitive use cases, to ensure the answer really reflects all relevant sources.
But this is where BLU DELTA’s expertise comes in: We develop systems that master these nuances — practical, scalable, and transparent.
Why BLU DELTA Relies on RAG
At BLU DELTA, we focus on technologies that don’t just impress — but deliver real value. RAG is a key concept for us to make AI solutions more accurate, transparent, and secure.
When combined with our expertise in OCR data extraction and process automation, it results in tremendous value: Intelligent systems that access your specific knowledge — whether digital, scanned, or automatically indexed.
One More Meta Note …
This blog article is, in a way, an example of RAG itself:
Information from a previously created internal PowerPoint presentation was “retrieved,” “augmented” with context, and “generated” into a coherent text.
The difference? This time, the process was done manually by a human inserting the document directly into the LLM chat. Making this process semi- or fully automated for your documents, data, and OCR content is our goal!
Want to Learn More About RAG?
Are you considering how to use RAG and OCR in your company — for example, to analyze invoices, contracts, or policies?
Then talk to us! The BLU DELTA team will be happy to advise you. Get in touch now!
BLU DELTA is a product for the automated capture of financial documents. Partners, but also finance departments, accounts payable accountants and tax advisors of our customers can use BLU DELTA to immediately relieve their employees of the time-consuming and mostly manual capture of documents by using BLU DELTA AI and Cloud.
BLU DELTA is an artificial intelligence from Blumatix Intelligence GmbH.
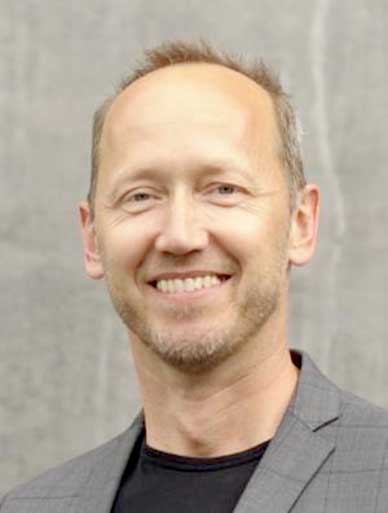
Author:Martin Loiperdinger is Co-Founder and CEO of Blumatix Intelligence GmbH. Previously, he was responsible for the development of copy protection solutions at an internationally operating corporation and later worked as an independent consultant for medium-sized companies and large enterprises. Since 2016, he has been driving AI-supported document processing, making Blumatix one of the most innovative providers in the DACH region. His goal is to enable seamless information exchange between companies.
Contact: m.loiperdinger@blumatix.at